In 2012, technologists Thomas H. Davenport and DJ Patil declared:
Data Scientist is the Sexiest job of the 21st Century (1)
A decade later, is Data Science still the sexiest job? or Is Data Science dying? What’s the state of Data Science in 2022 (2)?
To answer these questions, we investigate Anaconda’s (3) own industry survey conducted from April 25, 2022, to May 14, 2022 and visualize the results using PowerBI.
3,493 individuals who work in the Data Science field, from 133 countries and regions took part in this survey.
Demographic view of the Data Scientists in the world
Below are the demographics of the participants in the survey:
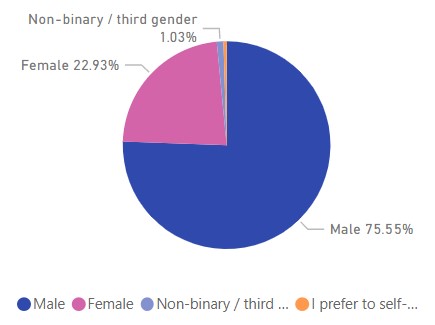
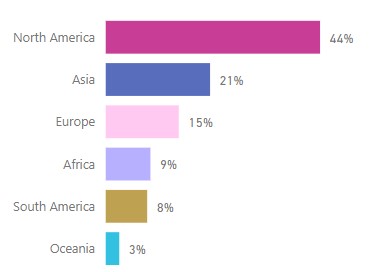
Most of the respondents are Male. We can say that the field of Data Science has a strong presence of Male (~76%), and much less data scientists are Female (~23%).
Survey respondents are present mostly in North America with 44%, 21% are present in Asia, 15% in Europe, 9% in Africa, 8% in South America, and 3% in Oceania.
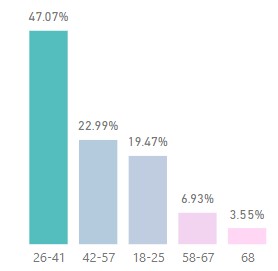
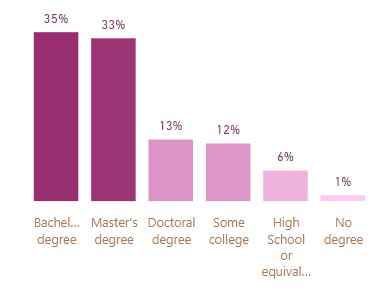
Nearly half of the data scientists (47%) are of age 26 to 41, and there’s no age limit for specialists in this field as we have data scientists in their 60s.
Data science is mainly taught in universities. 81% of respondents have a master’s, a bachelor’s, or a doctoral degree, and only a small percentage (1%) are self-taught.
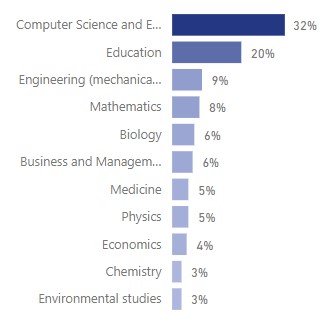
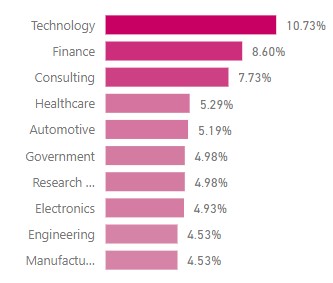
Data scientists come from different backgrounds, 32% come from a computer science and engineering background which is the largest percentage.
Data scientists work in industries related to technology mostly (10.73%), Finance (8.6%), consulting (7.73%), and healthcare (5.29%).
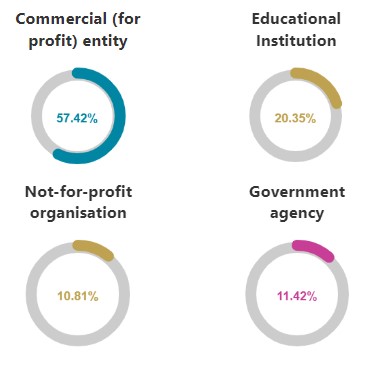
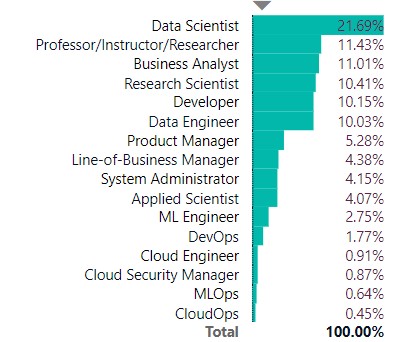
Commercial companies are the ones who employ the most significant number of data scientists (57.42%) in the world. Next is the educational institutions (20.5%). Government agencies and not-for-profit organizations employ the least number of data scientists (11%).
Data Science is a broad term. By Data Science we mean different roles in the companies:
The title Data Scientist is mainly used in organizations (21.69%), but other job titles have emerged, getting more specific: researchers/professors (11.43%), business analysts (11.01%), research engineers, research scientists, data engineers (10%), and many more.
What is Data Science all about? What tasks does it involve?
As said, there are different roles in Data Science. Depending on the role, tasks can vary between Data preparation, Data cleansing, Data visualization, Model selection, Model Training, Deploying models and Reporting and presentation.
Below is the percentage of time spent on each of the tasks for the roles of Data Scientist, Business Analyst, Data Engineer, Research scientist, ML Engineer and Applied scientist.
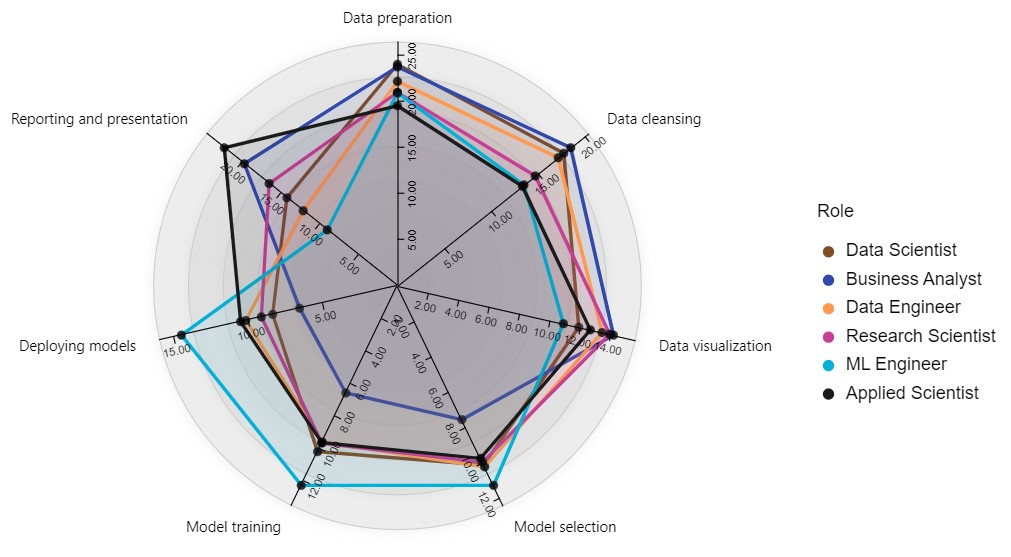
A person with a Data scientist role spends more time than others on data preparation and cleansing (38%).
An ML Engineer spends more time than others on Model Deploying, and Model Training, and spends the least time on Reporting and presentation.
We notice that data preparation and cleansing are important for all the roles in the Data Science field almost equally.
What most important skills missing in the Data Science/ML area in organizations?
Data Science is a mix of 5 categories of skills: Analytical skills, Business skills, Computing and Engineering skills, Data skills, and Maths skills.
Below are the skills missing in these categories, in percentages:
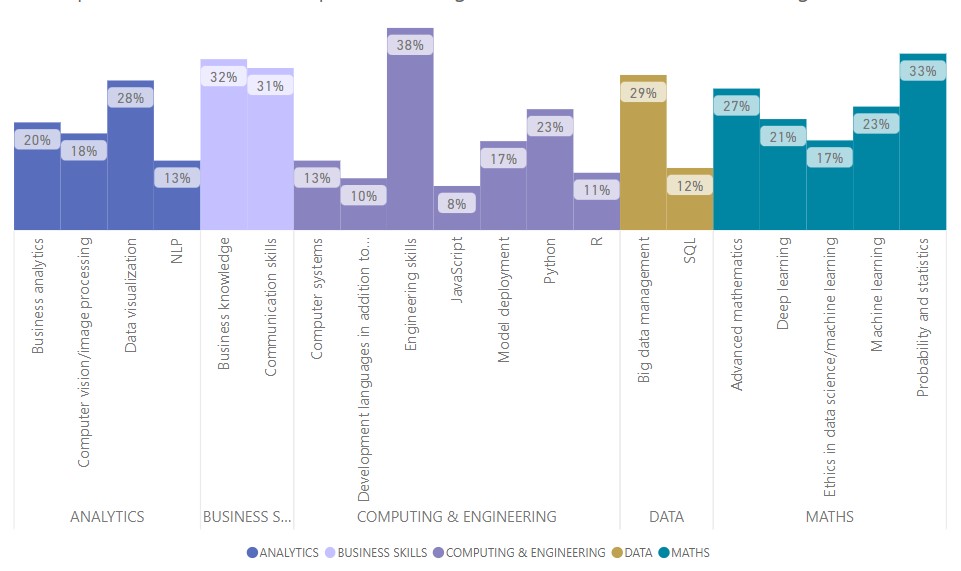
Maths, Computing, and engineering skills are the most missing skills in organizations, specifically, probability and statistics skills (33%), and engineering skills (38%) that include designing and implementing systems for collecting data, and storing and analyzing data at scale.
Therefore, there’s plenty of room for advancement in this field by getting more people to learn these missing skills.
What’s the biggest obstacle to obtaining the experience required for a career in data science?
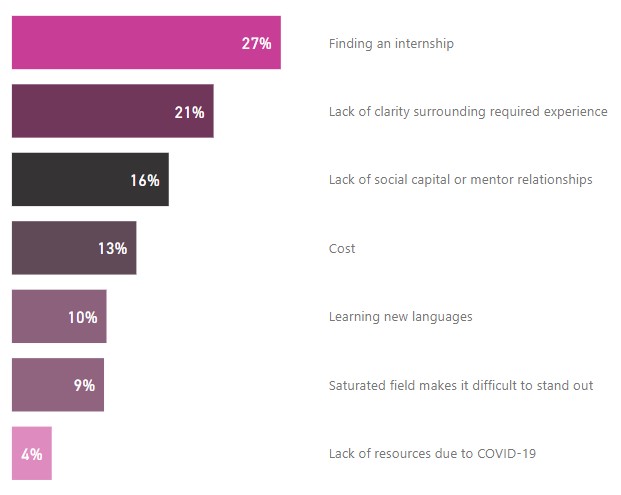
Data shows that finding an internship is the biggest issue for experience seekers (27%). 21% of respondents find that organizations aren’t clear about the experience required for the role. That is due to the many jobs that a data scientist can take.
Fig11: Obstacles faced by data scientists in percent
What are the languages required in organizations and how often are they used?
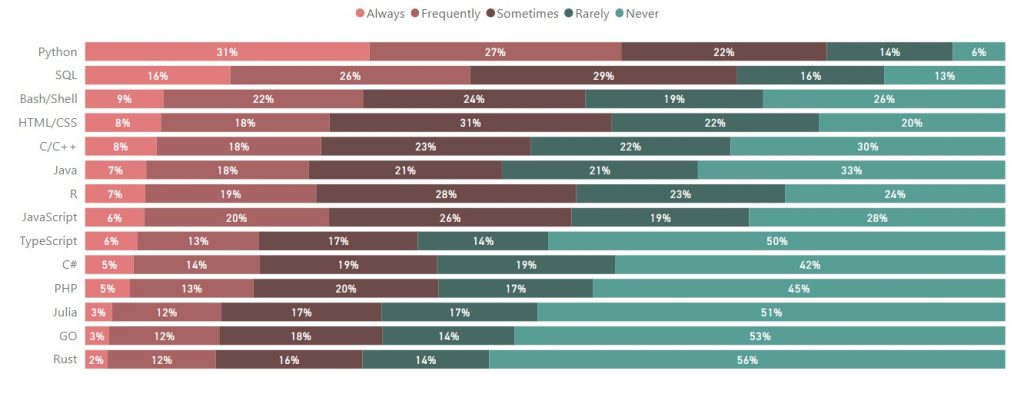
The majority (58%) of survey respondents indicated they always or frequently use Python. Only 6% of respondents never use Python. Which makes Python the most popular language in organizations.
SQL comes second (42%). It is still widely used in the community.
The graph below shows the importance of 14 different languages in Data Science.
What are the popular tools used in organizations and how often are they used?
In Data visualization:
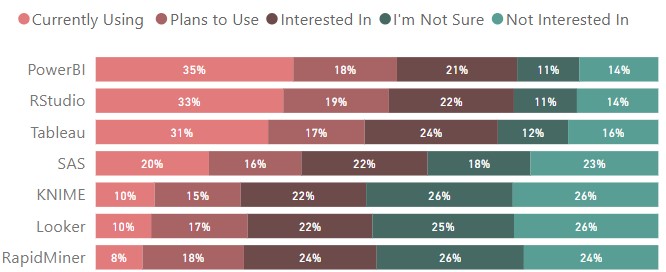
The most popular tool in Data visualization is Powerbi (53%) which organizations are currently using or planning to use. Rstudio is very close to PowerBI, and comes in second place with 52%.
Fig13: Usage of Dataviz tools in percent
In Repositories:
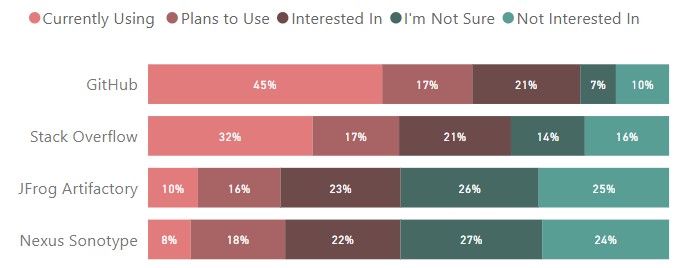
The most popular tool in Repositories is GitHub (62%) which organizations are currently using or planning to use. comes in second place Stack Overflow (49%).
Fig14: Usage of Repositories in percent
In ML Platforms:
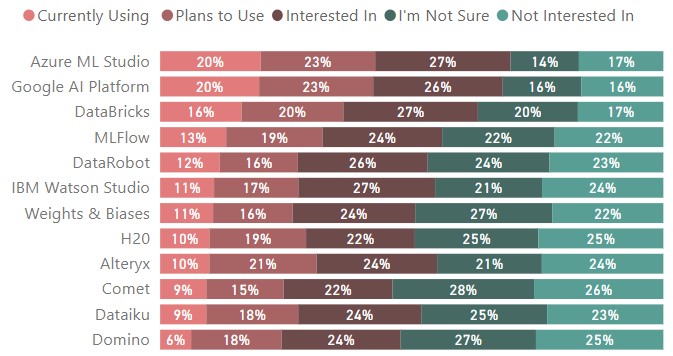
The most popular tool in ML Platforms is equally Azure ML Studio and Google AI Platform (43%) which organizations are currently using or planning to use.
Fig15: Usage of ML Platforms in percent
In Cloud Data Warehouses:

The most popular tool in Cloud Data Warehouses is Google BigQuery (42%) which organizations are currently using or planning to use.
Fig16: Usage of Cloud Data Warehouses tools in percent
In Data Science platforms:
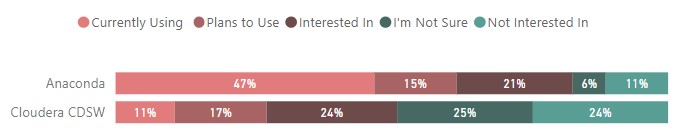
The most popular tool in DS Platforms is Anaconda (62%) which organizations are currently using or planning to use. It comes before Cloudera CDSW (28%).
Fig17: Usage of Data Science platforms in percent
Conclusion and recommendation
In summary, Data Science is a wide field that has a strong male presence, and mostly in the North of America. It’s popular between age groups 26 to 41, and has no limits on age. To become a data scientist, one need a university degree as it can ease the process of learning the craft. Computer science and engineering are the most common backgrounds for data scientists. Although data science is present in many industries, commercial companies in industries related to technology embrace most data scientists.
Data Science is almost an umbrella term with a wide range of functions. 10 years ago and even today, different people will tell us what Data Science means differently.
As companies start to come to a consensus on what Data Science can really do for them, that’s when more specific and specialized job functions emerge under the Data Science umbrella.
Soon there will be nobody with the title Data Scientist in organizations, as we can see that organizations have started to have more specified titles such as Data engineers, Research scientists, and Decision scientists.
Data preparation and cleansing are the most time consuming tasks regardless of the title in Data science.
On another hand, there are many missing skills. Universities need to be updated on the organizations’ needs of skills in the Data Science field and thus direct their students into learning these skills, especially maths, computing and engineering.
Python from Anaconda is used widely in organizations and is expected to continue to be. More tools will continue to be developped in the many fields of data science.
Data Science is not dying. It’s evolving and it’s just getting started.
Data source:
- (1) https://hbr.org/2012/10/data-scientist-the-sexiest-job-of-the-21st-century
- (2) https://www.anaconda.com/state-of-data-science-report-2022
- (3) Anaconda is the distribution of the Python and R programming languages for scientific computing, widely used in the community of data scientists
Data Scientists are booming the market today!! Good choice and very insightful to publish and to visualize!